Annotation Services
At Outline Media Solutions, our Annotation Services are crafted to bring clarity and precision to your data,
setting a solid foundation for machine learning and AI models across industries.
With our specialized annotation techniques, we help you unlock the potential of your visual data, enhancing accuracy and insight.
Bounding Box Annotation
Bounding Box Annotation is a fundamental technique used to capture and label objects in images and videos, framing them in rectangles to make detection simpler and more efficient.
- Object Tracking: Seamlessly follow and label objects across frames, ensuring that each entity is consistently recognized. This service is essential for applications like autonomous vehicles, where understanding movement and tracking objects over time ensures safety and reliability.
- Image Annotation for Retail and E-commerce: Empower e-commerce platforms with well-defined, labeled images that enhance search algorithms and product classification. By annotating products precisely, we help improve image-based search accuracy and facilitate easy browsing experiences for customers.
- Object Detection: Our bounding box annotations allow machines to quickly identify essential objects in various environments, such as pedestrians, vehicles, animals, or stationary obstacles. This capability is especially critical for applications in autonomous navigation and real-time surveillance.
Polygon Annotation
Polygon Annotation provides exact outlines for objects with irregular shapes,
allowing models to capture fine details that rectangular boxes can’t encompass.
Polygon Image Annotation for Semantic Segmentation
We label complex shapes at a pixel level to categorize every part of an image. Ideal for projects that require high-detail labeling, such as in medical imaging, urban planning, and environmental studies, where precision is essential to accurately identifying different regions.
Object Detection for Aerial Views
Using polygon annotation, we help identify buildings, road networks, and natural features from aerial perspectives. This service is valuable for geographical information systems (GIS), satellite imagery analysis, and environmental research where object shape and placement are crucial.
Polygon Shapes for the Agriculture Industry
By using polygon annotations, we offer precise outlines of crops, soil patches, and other field areas, which helps agricultural AI applications assess crop health, land usage, and environmental factors.
Autonomous Vehicle Annotation
Our autonomous vehicle annotation services are designed to provide the most relevant and accurate
data needed for safe and effective navigation in real-world environments.
- Road and Lane Marking: Our team annotates lanes, road boundaries, and traffic signs to ensure vehicles can “see” the road ahead. Accurate lane and road marking annotation is vital for safe lane-keeping, lane-switching, and maneuvering.
- Pedestrian and Object Detection: We label pedestrians, cyclists, other vehicles, and potential obstacles to help autonomous systems respond intelligently to their surroundings. This service ensures that autonomous vehicles can distinguish between dynamic and static objects, supporting safe decision-making on the road.
- Traffic Sign and Light Recognition: Annotate traffic signs, lights, and road markings for precise identification and compliance with local road rules. Our annotations make it possible for autonomous systems to navigate complex environments with confidence.
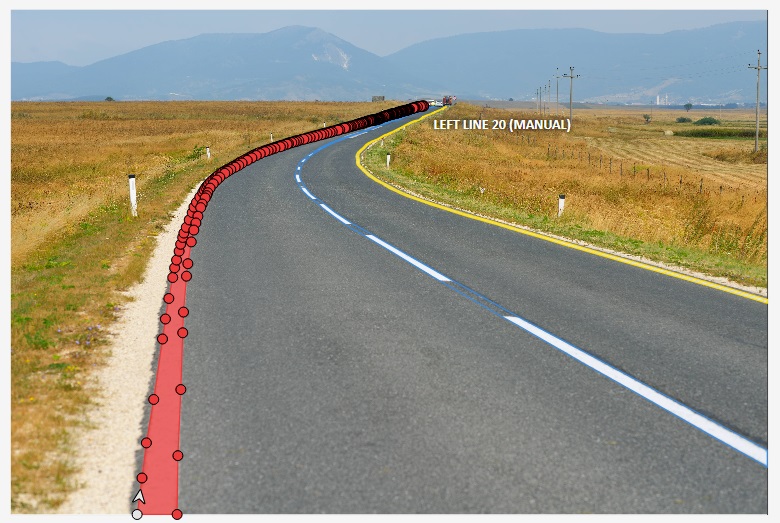
3D Cuboid Annotation
3D Cuboid Annotation brings depth and spatial awareness to annotations,
creating a multi-dimensional perspective essential for applications in robotics, AR/VR, and autonomous driving.
3D Object Detection
Mark objects with cuboids that define their dimensions in three-dimensional space. This service helps models gauge object placement, distance, and orientation, crucial for autonomous vehicles that need to anticipate and respond to objects in their path.
Object Orientation and
Distance Measurement
We annotate object orientation and measure distances to improve the spatial understanding of AI models. This is especially useful for drone navigation, robotic handling, and augmented reality applications where depth perception is key.
Image Annotation and Labeling
Image Annotation and Labeling enrich visual data by tagging and categorizing objects, scenes, and attributes. Our labeling provides a deeper understanding of each visual element, making it easy to interpret and utilize for machine learning.
Class-Based Labeling
Our team categorizes objects by class, helping models to understand distinctions between object types such as animals, vehicles, or products. This labeling approach enhances generalization across multiple use cases, enabling accurate recognition in real-world applications.
Attribute Annotation
Add layers of information with attribute-based labeling, specifying features like color, texture, size, or condition. This enables highly targeted applications, such as in retail, where details on attributes improve product searches and recommendations.
Landmark Annotation
Mark specific points, like facial landmarks or anatomical features, to facilitate detailed analysis in areas such as healthcare, facial recognition, and human motion studies.
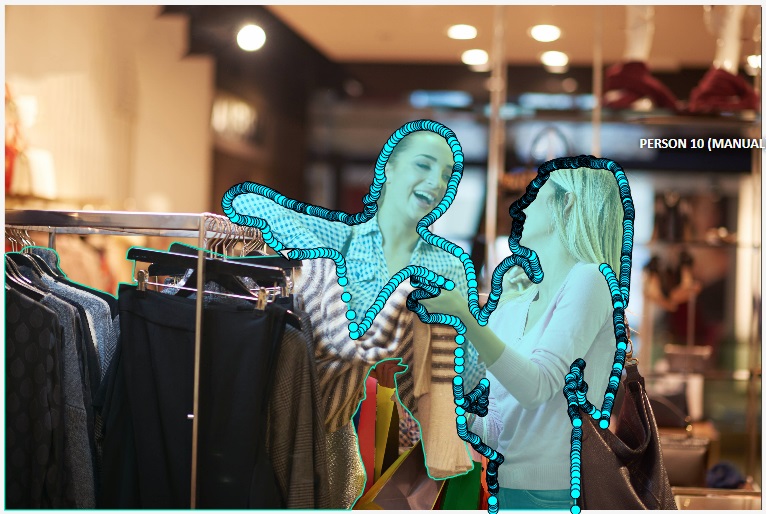
Video Annotation
Video Annotation involves capturing and labeling data across multiple frames,
making it possible to analyze movements, behaviors, and scene changes over time.
Frame-by-Frame Object Tracking
Our experts annotate objects across each frame, ensuring consistent labeling for seamless tracking. This service is crucial for video-based AI applications, from autonomous vehicles to security monitoring and sports analytics.
Action and Behavior
Recognition
We identify and label specific actions, such as walking, running, or driving, adding an extra layer of context to the data. This is ideal for use in behavioral analysis, sports analytics, and surveillance systems where understanding movement is key.
Scene Analysis and Contextual Tagging
Our annotations provide comprehensive scene analysis, capturing and tagging contextual elements that help AI models understand the broader environment, ideal for autonomous navigation and situational awareness in AI systems.